👋 Hoi!
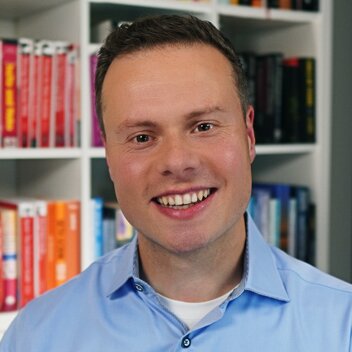
💻 I work at Xomnia in Amsterdam as a Senior Machine Learning Engineer.
✍️ I’m currently writing Python Polars: The Definitive Guide with Thijs Nieuwdorp. I’m also the author of Data Science at the Command Line. The second edition was published by O’Reilly in 2021.
🐛 Most of my bugs are written in Python, R, JavaScript and Bash. Every now and then I dabble in C and Lua. I should learn Rust.
🇳🇱 I live in Rotterdam, the Netherlands with my wife and two kids.
🗽 My wife and I have lived in New York City for 2,5 years. I try to visit this beautiful place at least once a year.
🪚 I’m building a Lego table in my spare time. Still got all my fingers.
📼 I’ve seen Labyrinth (1986) and The Matrix (1999) more times than I care to count.
Latest articles
- Dimensionality Reduction, Animated
- Plotnine: Grammar of Graphics for Python
- We Are Writing Python Polars: The Definitive Guide
Newsletter
Would you like to receive an email whenever I have a new blog post, organize an event, or have an important announcement to make? Sign up to my newsletter: